Machine-learning-derived heart and brain age
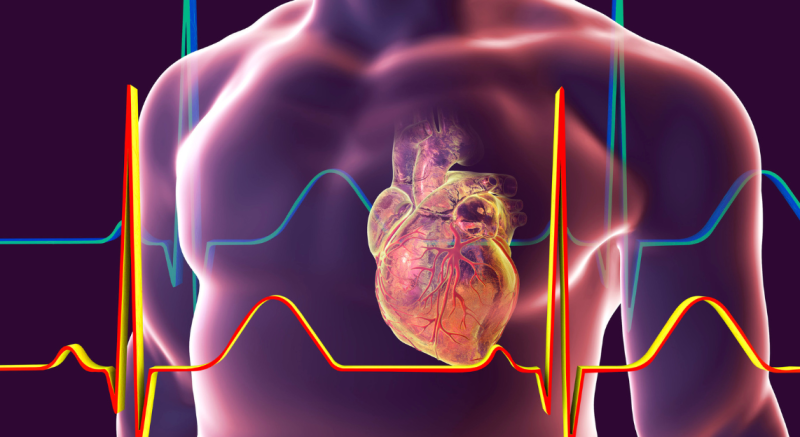
The difference between actual chronological age and a biological age that are predicted using artificial intelligence based on electrocardiograms can describe an individual’s physiological deterioration and hence can be used as a marker of physical health. This biomarker may be used as a component of clinical risk prediction scores as well as to shed insight upon more fundamental aspects of biological ageing.
Project period: 03.01.2022 - 01.08.2025
Cardiovascular event rates increase with age in all populations. This is thought to be the result of multiple underlying molecular and cellular processes that lead to a process of vascular ageing. Apart from arterial stiffness based on pulse wave velocity there are almost no other non-invasive measures of vascular ageing. Recently a novel biomarker of vascular ageing has been proposed based on the application of a deep-learning algorithm to digitised 12-lead electrocardiograms (ECG). Having a predicted ECG-age that is greater than chronological age has been shown to be associated with an adverse CVD risk factor profile and higher mortality relative to predicted ECG-age being lower than chronological age. In principle this biomarker holds the promise of being able to be used as a component of clinical risk prediction scores as well as throwing light upon more fundamental aspects of biological ageing. However, much is still unknown about the determinants of this difference between ECG-age and chronological age (δ-age) and how far it is predictive of age-related frailty that goes beyond cardiovascular disease per se. The objective of this project is to harness data collected over various waves of the Tromsø Study and explore in depth the properties of the novel biomarker δ-age in order to better assess its potential value as an index of the rate of vascular / cardiovascular system ageing and more broadly of other aspects of the ageing phenotype.
Specific aims:
- What are the prospective associations between CVD risk factors and biomarkers (blood pressure, heart rate, blood lipids, diabetes, BMI, waist to hip ratio, high sensitivity C-reactive protein) and δ-age?
- What is the association between socio-economic position and δ-age and how far is it mediated by measured biological and behavioural risk factors?
- What are the cross-sectional associations between markers of physical function and δ-age?
- What are the cross-sectional associations between cognitive function and δ-age and brain age?
- Does δ-age calculated using deep neural networks trained in different populations have the same ability to predict onset of and mortality due to cardiovascular disease, dementia, cancer, diabetes, chronic obstructive pulmonary disease?
Members:
Tom Wilsgaard (Principal investigator)
Olena Iakunchykova, UiO
David Leon, LSHTM
Arya Panthalanickal Vijayakumar
Anne Elise Eggen
Inger Njølstad
Henrik Schirmer, UiO
Haakon Lindekleiv
Francisco Lopez-Jimenez, Mayo Clinic
Torgil Vangberg
Publications
Financial/grant information:
HNF1636-22, grant from Northern Norway Regional Health Authority